Why Should You Use Sum Instead of Average in Your Calculations?
In the world of data analysis and statistics, the choice between using the sum or the average can significantly influence the interpretation of results. While averages are often hailed for their simplicity and ease of understanding, there are instances where relying on the sum can provide a more accurate and insightful picture of the data at hand. This article delves into the nuances of these two fundamental concepts, exploring the contexts in which using the sum instead of the average can lead to more meaningful conclusions.
Understanding the distinction between sum and average is crucial for anyone working with numerical data. The sum represents the total of all values, while the average provides a central value that may not fully capture the distribution or variability within the dataset. In certain scenarios, particularly when dealing with skewed data or outliers, the sum can highlight trends and patterns that averages might obscure. For instance, in financial analysis, total revenue or expenses can reveal the scale of operations, while averages might mislead stakeholders about performance.
Moreover, the implications of choosing one metric over the other extend beyond mere calculations; they can affect decision-making processes and strategic planning. By examining case studies and practical applications, we can uncover why analysts and researchers may opt for the sum in specific situations, ultimately leading to a more nuanced understanding of data interpretation. Join us as
Understanding the Difference Between Sum and Average
In data analysis, the choice between using sum or average can significantly impact the interpretation of results. The sum is the total obtained by adding all the individual values in a dataset, while the average is calculated by dividing the sum by the number of values. Each measure serves distinct purposes and has different implications for data interpretation.
- Sum: Provides a total value, useful for understanding the overall volume or size of a dataset.
- Average: Offers a centralized value that represents a dataset, allowing for comparisons between different datasets or subsets.
When to Use Sum Instead of Average
Using sum instead of average can be advantageous in several scenarios:
- Aggregating Total Contributions: When assessing total sales, expenses, or any cumulative metric, using the sum provides a clear picture of overall performance.
- Understanding Proportions: In cases where proportions or ratios are needed, the sum helps to calculate these metrics accurately.
- Variability in Data Size: If datasets vary significantly in size, using the average can lead to misleading conclusions. The sum maintains the actual total regardless of the number of observations.
Practical Examples
Consider a company analyzing sales data for two products over three months:
Product | Month 1 | Month 2 | Month 3 | Total Sales | Average Sales |
---|---|---|---|---|---|
Product A | 500 | 700 | 800 | 2000 | 666.67 |
Product B | 300 | 400 | 500 | 1200 | 400 |
In this example, the total sales for Product A is more significant than Product B. If only the average were considered, it might mislead analysts into thinking both products performed similarly when they did not.
Considerations for Choosing Between Sum and Average
When deciding whether to use sum or average, consider the following factors:
- Nature of Data: For continuous variables, averages may be more relevant, while sums are critical for discrete data.
- Presence of Outliers: Averages can be skewed by extreme values, while sums remain unaffected, making them more reliable in certain scenarios.
- Audience Understanding: Depending on the audience’s familiarity with the data, sums might convey more straightforward insights, whereas averages might require additional context.
In summary, while averages can provide insights into general trends, sums deliver essential information about total quantities, guiding more informed decision-making in various analytical contexts.
Understanding the Use of Sum Instead of Average
In statistical analysis, the choice between using a sum and an average often depends on the context and the specific insights one aims to derive from the data. Understanding the implications of using the sum instead of the average can lead to more accurate interpretations and informed decisions.
When to Use Sum Over Average
There are specific scenarios where utilizing the sum provides a clearer picture of the data:
- Total Quantification: When the overall quantity is needed, such as total sales, total expenses, or total population in a study.
- Data Aggregation: In cases where aggregating the raw data is crucial, such as calculating total revenue for a business over a fiscal year.
- Non-normalized Data: When the data set is small or when the scale of measurement varies significantly, the sum can provide a more meaningful representation than the average.
Advantages of Using Sum
- Clarity in Reporting: The sum can provide a straightforward narrative of the total without the need for further calculations, making it easier for stakeholders to grasp the overall figures.
- Avoids Misleading Averages: Averages can sometimes obscure the reality of the data set, particularly in cases with outliers or skewed distributions. The sum maintains the integrity of the data.
- Facilitates Comparison: When comparing different groups or categories, sums allow for direct comparisons of total values rather than relying on potentially misleading averages.
Disadvantages of Using Sum
- Lack of Context: The sum alone does not provide insight into the distribution or variability of the data, which can be essential for comprehensive analysis.
- Inapplicability in Certain Analyses: In statistical tests or models where normal distribution is assumed, using an average may be necessary for valid conclusions.
- Scalability Issues: Summing large datasets without normalizing can lead to misinterpretations, especially in populations with differing sizes.
When to Prefer Average
In contrast, there are circumstances where the average may be more appropriate:
- Standardization: Averages facilitate comparisons across different categories or time periods by normalizing data.
- Insight into Trends: Averages can reveal trends over time, especially in longitudinal studies where changes in behavior or metrics are analyzed.
- Robustness to Outliers: In datasets with extreme values, averages can provide a central point of reference that is less affected by those extremes than the sum.
Example Comparison
Consider a scenario with sales data from three stores:
Store | Sales ($) |
---|---|
A | 100 |
B | 200 |
C | 1,000 |
- Sum: $100 + $200 + $1,000 = $1,300
- Average: $1,300 / 3 = $433.33
In this case, the sum highlights the total sales, while the average may misrepresent the performance of individual stores, particularly Store C, which greatly skews the average.
Conclusion on Usage
The decision to use sum or average should be guided by the analytical objectives and the nature of the data. Both metrics serve unique purposes and can be employed in tandem for a more nuanced understanding of the data set. Employing both can provide a comprehensive view, ensuring that analyses are both thorough and insightful.
Evaluating the Use of Sum Over Average in Data Analysis
Dr. Emily Chen (Data Scientist, Analytics Innovations). The choice between using sum and average in data analysis hinges on the specific insights one aims to derive. While averages provide a general overview, sums can reveal total impact and variations that averages may obscure. In scenarios where total performance or cumulative effects are critical, opting for sum is often more informative.
Michael Thompson (Financial Analyst, Market Insights Group). In financial modeling, using sum instead of average can significantly alter the interpretation of data trends. For instance, when assessing total revenue or expenses, the sum offers a clearer picture of overall financial health, whereas averages can dilute the impact of outliers or extreme values that are crucial for strategic decisions.
Sarah Patel (Statistical Consultant, Data Solutions Inc.). It is essential to consider the context of the data when choosing between sum and average. In many cases, the sum provides a more accurate representation of the total dataset, especially in areas like resource allocation or population studies, where understanding the total count or volume is vital for effective decision-making.
Frequently Asked Questions (FAQs)
Why would someone use the sum instead of the average?
Using the sum instead of the average can provide a clearer picture of total values, especially in contexts where the aggregate amount is more relevant than the per-unit measure. This is particularly useful in financial analysis, budgeting, and resource allocation.
In what scenarios is it more appropriate to use the sum?
It is more appropriate to use the sum in situations where total quantities are needed, such as calculating total sales, expenses, or population counts. Additionally, when assessing cumulative data over time, the sum offers a more comprehensive view.
Can using the sum lead to misinterpretation of data?
Yes, relying solely on the sum can lead to misinterpretation, especially if the context requires understanding averages or rates. Without considering the number of units involved, stakeholders may overlook important trends or patterns.
How does using the sum affect data analysis?
Using the sum can enhance data analysis by highlighting total impacts and trends. However, it may also obscure individual variations and lead to conclusions that do not account for the distribution of data points.
Are there specific fields where using the sum is preferred over the average?
Yes, fields such as finance, economics, and project management often prefer the sum for total revenue, costs, or resource utilization. In these contexts, understanding the overall impact is crucial for decision-making.
What is a common mistake when using the sum instead of the average?
A common mistake is failing to provide context for the sum, such as the number of items or time period involved. This can mislead stakeholders about the significance of the total and its implications for performance or outcomes.
The discussion surrounding the use of the sum in place of the average highlights a fundamental difference in how data can be interpreted and utilized in various contexts. The sum provides a total value that can be particularly useful in scenarios where the overall quantity is more relevant than the individual contributions. For instance, in financial analysis, understanding total revenue or expenses can offer clearer insights into a company’s performance than merely looking at average figures. This approach can often reveal trends and patterns that averages may obscure.
Moreover, using the sum instead of the average can be advantageous in specific analytical frameworks, such as when dealing with skewed data distributions. In cases where data points are heavily concentrated around certain values, the average may not accurately represent the dataset’s characteristics. Relying on the sum allows analysts to consider the total impact of all data points, leading to more informed decision-making processes. This is particularly evident in fields like economics and social sciences, where aggregate data can drive policy decisions.
while averages serve as a useful metric in many situations, the sum provides a vital alternative that can enhance data analysis. It is essential to consider the context and objectives of the analysis when choosing between these two measures. By understanding the implications of using the sum instead of the average
Author Profile
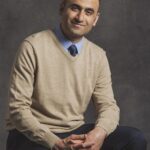
-
Dr. Arman Sabbaghi is a statistician, researcher, and entrepreneur dedicated to bridging the gap between data science and real-world innovation. With a Ph.D. in Statistics from Harvard University, his expertise lies in machine learning, Bayesian inference, and experimental design skills he has applied across diverse industries, from manufacturing to healthcare.
Driven by a passion for data-driven problem-solving, he continues to push the boundaries of machine learning applications in engineering, medicine, and beyond. Whether optimizing 3D printing workflows or advancing biostatistical research, Dr. Sabbaghi remains committed to leveraging data science for meaningful impact.
Latest entries
- March 22, 2025Kubernetes ManagementDo I Really Need Kubernetes for My Application: A Comprehensive Guide?
- March 22, 2025Kubernetes ManagementHow Can You Effectively Restart a Kubernetes Pod?
- March 22, 2025Kubernetes ManagementHow Can You Install Calico in Kubernetes: A Step-by-Step Guide?
- March 22, 2025TroubleshootingHow Can You Fix a CrashLoopBackOff in Your Kubernetes Pod?